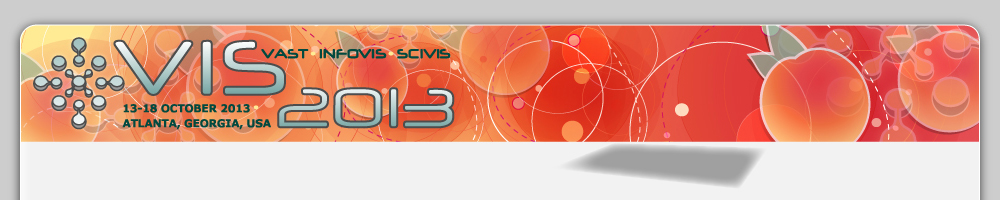
Spectral clustering is a powerful and versatile technique, whose broad range of applications includes 3D image analysis. However, its practical use often involves a tedious and time-consuming process of tuning parameters and making application-specific choices. In the absence of training data with labeled clusters, help from a human analyst is required to decide the number of clusters, to determine whether hierarchical clustering is needed, and to define the appropriate distance measures, parameters of the underlying graph, and type of graph Laplacian. We propose to simplify this process via an open-box approach, in which an interactive system visualizes the involved mathematical quantities, suggests parameter values, and provides immediate feedback to support the required decisions. Our framework focuses on applications in 3D image analysis, and links the abstract high-dimensional feature space used in spectral clustering to the three-dimensional data space. This provides a better understanding of the technique, and helps the analyst predict how well specific parameter settings will generalize to similar tasks. In addition, our system supports filtering outliers and labeling the final clusters in such a way that user actions can be recorded and transferred to different data in which the same structures are to be found. Our system supports a wide range of inputs, including triangular meshes, regular grids, and point clouds. We use our system to develop segmentation protocols in chest CT and brain MRI that are then successfully applied to other datasets in an automated manner.