GeoLinter: A Linting Framework for Choropleth Maps
Fan Lei -
Arlen Fan -
Alan M. MacEachren -
Ross Maciejewski -
Screen-reader Accessible PDF
Download preprint PDF
DOI: 10.1109/TVCG.2023.3322372
Room: Bayshore II
Wednesday, October 16th, 2024 @ 17:45GMT+00:00Change your timezone on the schedule page
6 months agoYour current time: Sunday, Apr 6th @ 09:12
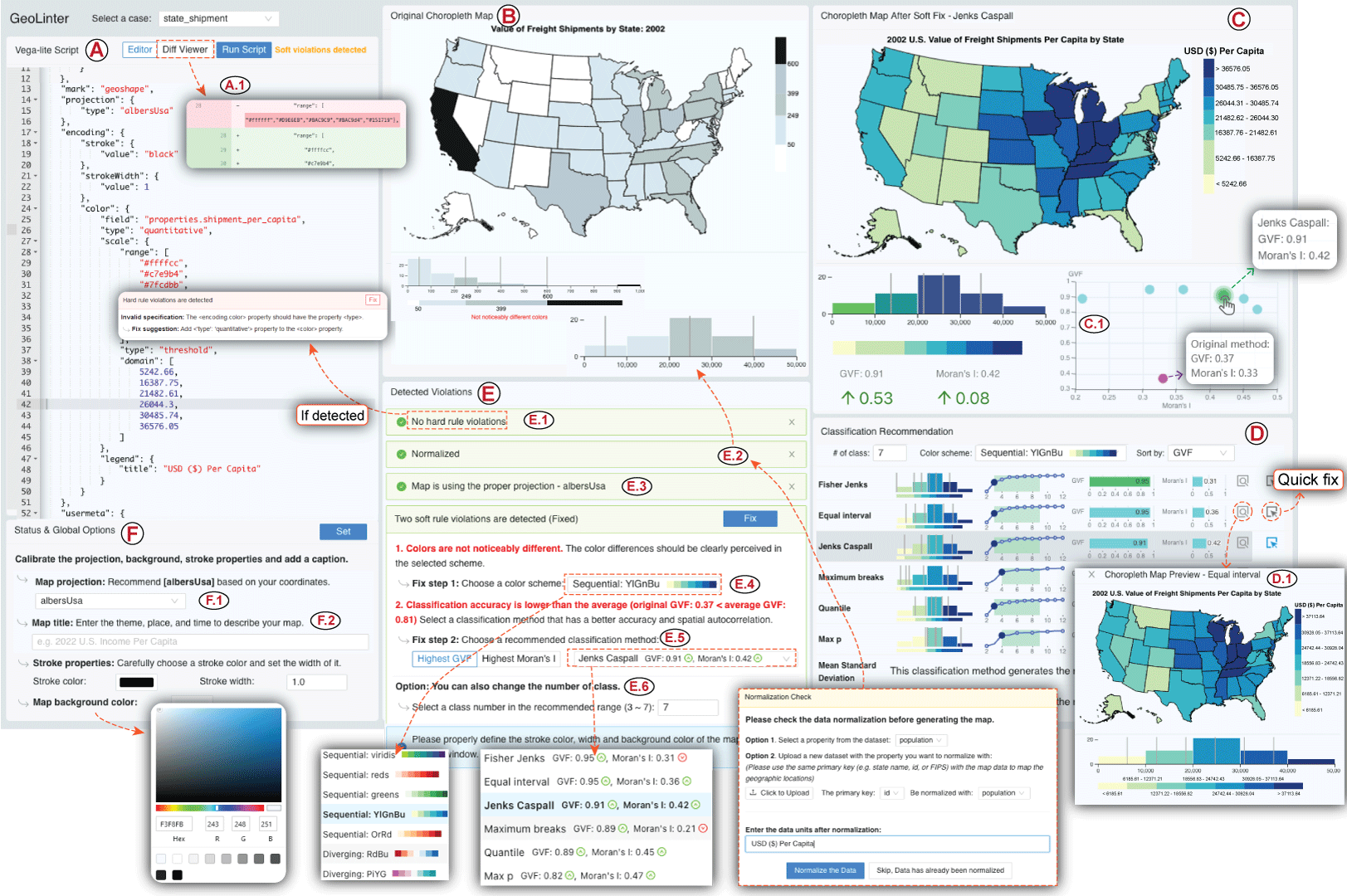
Fast forward
Full Video
Keywords
Data visualization , Image color analysis , Geology , Recommender systems , Guidelines , Bars , Visualization Author Keywords: Automated visualization design , choropleth maps , visualization linting , visualization recommendation
Abstract
Visualization linting is a proven effective tool in assisting users to follow established visualization guidelines. Despite its success, visualization linting for choropleth maps, one of the most popular visualizations on the internet, has yet to be investigated. In this paper, we present GeoLinter, a linting framework for choropleth maps that assists in creating accurate and robust maps. Based on a set of design guidelines and metrics drawing upon a collection of best practices from the cartographic literature, GeoLinter detects potentially suboptimal design decisions and provides further recommendations on design improvement with explanations at each step of the design process. We perform a validation study to evaluate the proposed framework's functionality with respect to identifying and fixing errors and apply its results to improve the robustness of GeoLinter. Finally, we demonstrate the effectiveness of the GeoLinter - validated through empirical studies - by applying it to a series of case studies using real-world datasets.