Uniform Sample Distribution in Scatterplots via Sector-based Transformation
Hennes Rave - University of Münster, Münster, Germany
Vladimir Molchanov - University of Münster, Münster, Germany
Lars Linsen - University of Münster, Münster, Germany
Room: Bayshore VI
2024-10-16T13:15:00ZGMT-0600Change your timezone on the schedule page
2024-10-16T13:15:00Z
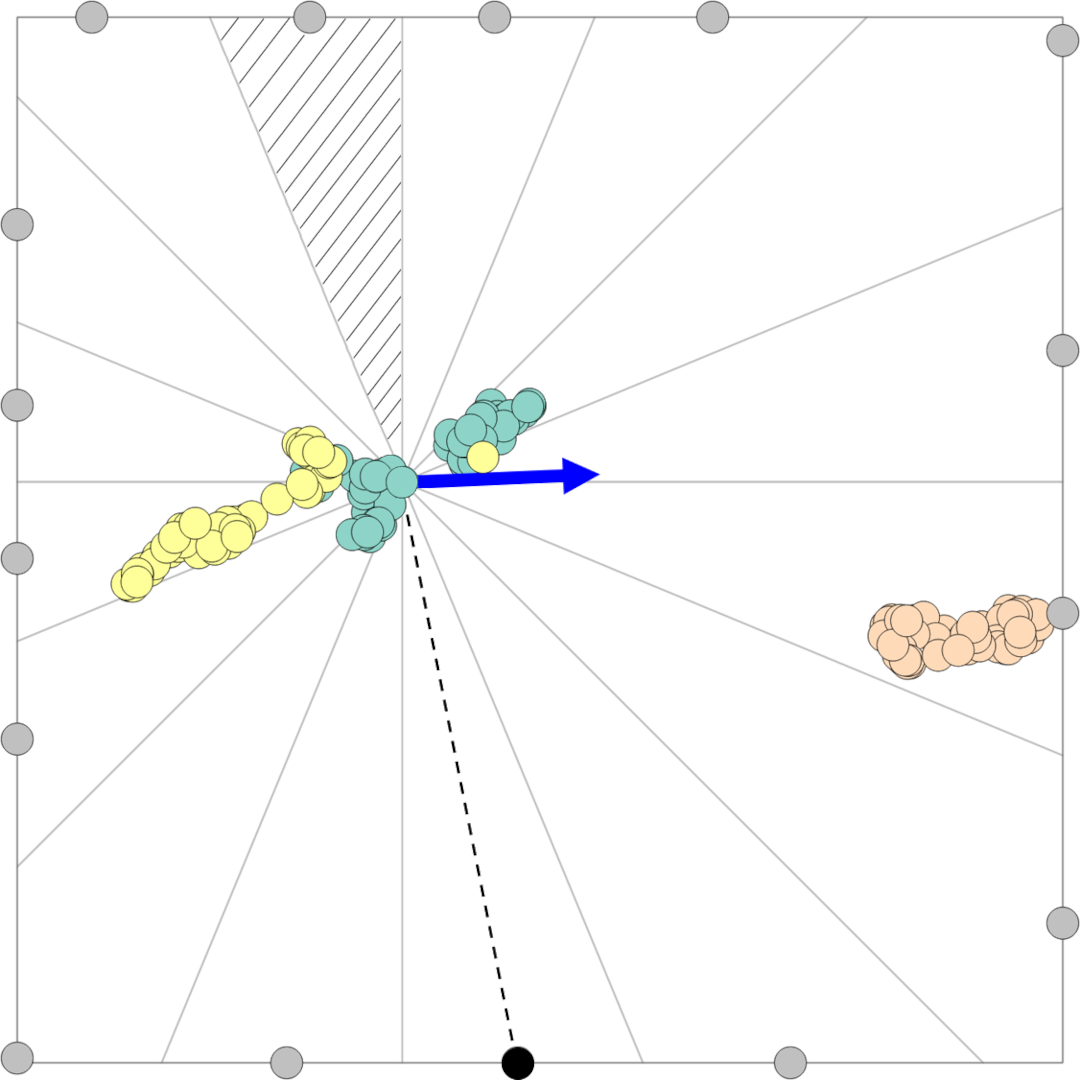
Fast forward
Full Video
Keywords
Scatterplot de-cluttering, spatial transformation.
Abstract
A high number of samples often leads to occlusion in scatterplots, which hinders data perception and analysis. De-cluttering approaches based on spatial transformation reduce visual clutter by remapping samples using the entire available scatterplot domain. Such regularized scatterplots may still be used for data analysis tasks, if the spatial transformation is smooth and preserves the original neighborhood relations of samples. Recently, Rave et al. proposed an efficient regularization method based on integral images. We propose a generalization of their regularization scheme using sector-based transformations with the aim of increasing sample uniformity of the resulting scatterplot. We document the improvement of our approach using various uniformity measures.