Honorable Mention
Guided Health-related Information Seeking from LLMs via Knowledge Graph Integration
Youfu Yan - University of Minnesota, Minneapolis, United States
Yu Hou - University of Minnesota, Minneapolis, United States
Yongkang Xiao - University of Minnesota, Minneapolis, United States
Rui Zhang - University of Minnesota, Minneapolis, United States
Qianwen Wang - University of Minnesota, Minneapolis , United States
Download preprint PDF
Download Supplemental Material
Room: Bayshore V
Friday, October 18th, 2024 @ 13:30GMT+00:00Change your timezone on the schedule page
6 months agoYour current time: Thursday, May 1st @ 17:39
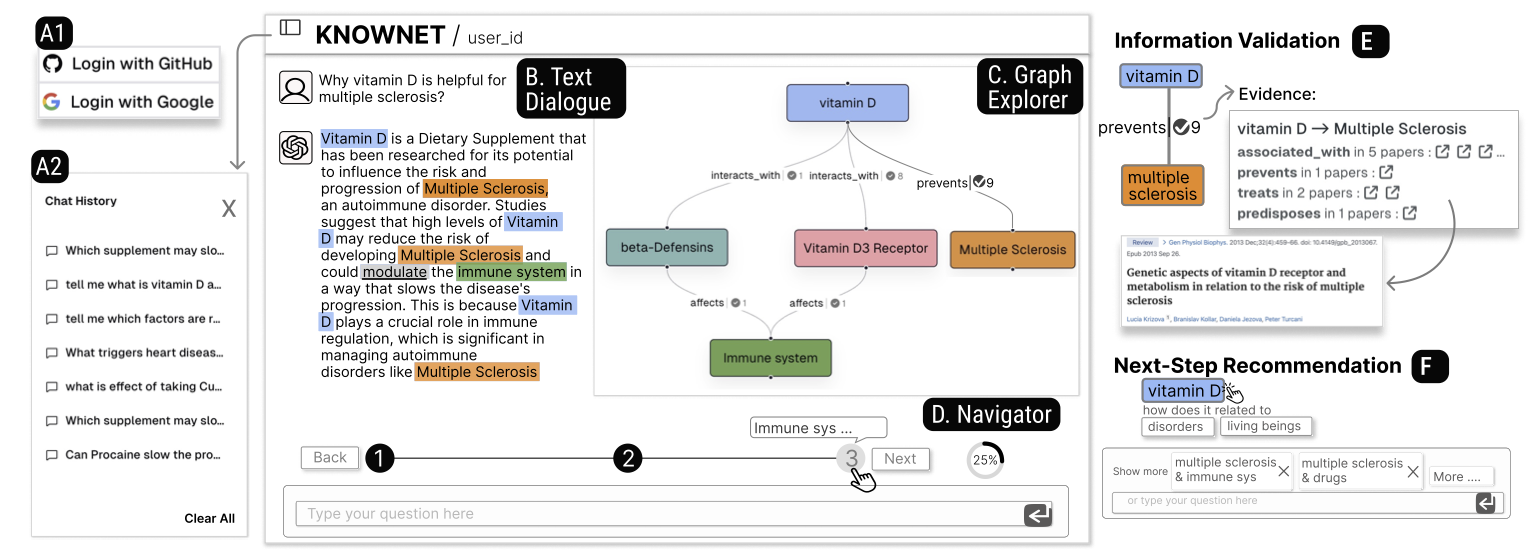
Fast forward
Full Video
Keywords
Human-AI interactions, knowledge graph, conversational agent, large language model, progressive visualization
Abstract
The increasing reliance on Large Language Models (LLMs) for health information seeking can pose severe risks due to the potential for misinformation and the complexity of these topics. This paper introduces KnowNet, a visualization system that integrates LLMs with Knowledge Graphs (KG) to provide enhanced accuracy and structured exploration. One core idea in KnowNet is to conceptualize the understanding of a subject as the gradual construction of graph visualization, aligning the user's cognitive process with both the structured data in KGs and the unstructured outputs from LLMs. Specifically, we extracted triples (e.g., entities and their relations) from LLM outputs and mapped them into the validated information and supported evidence in external KGs. Based on the neighborhood of the currently explored entities in KGs, KnowNet provides recommendations for further inquiry, aiming to guide a comprehensive understanding without overlooking critical aspects. A progressive graph visualization is proposed to show the alignment between LLMs and KGs, track previous inquiries, and connect this history with current queries and next-step recommendations. We demonstrate the effectiveness of our system via use cases and expert interviews.