Promises and Pitfalls: Using Large Language Models to Generate Visualization Items
Yuan Cui - Northwestern University, Evanston, United States
Lily W. Ge - Northwestern University, Evanston, United States
Yiren Ding - Worcester Polytechnic Institute, Worcester, United States
Lane Harrison - Worcester Polytechnic Institute, Worcester, United States
Fumeng Yang - Northwestern University, Evanston, United States
Matthew Kay - Northwestern University, Chicago, United States
Download preprint PDF
Download Supplemental Material
Room: Bayshore I + II + III
2024-10-18T13:06:00ZGMT-0600Change your timezone on the schedule page
2024-10-18T13:06:00Z
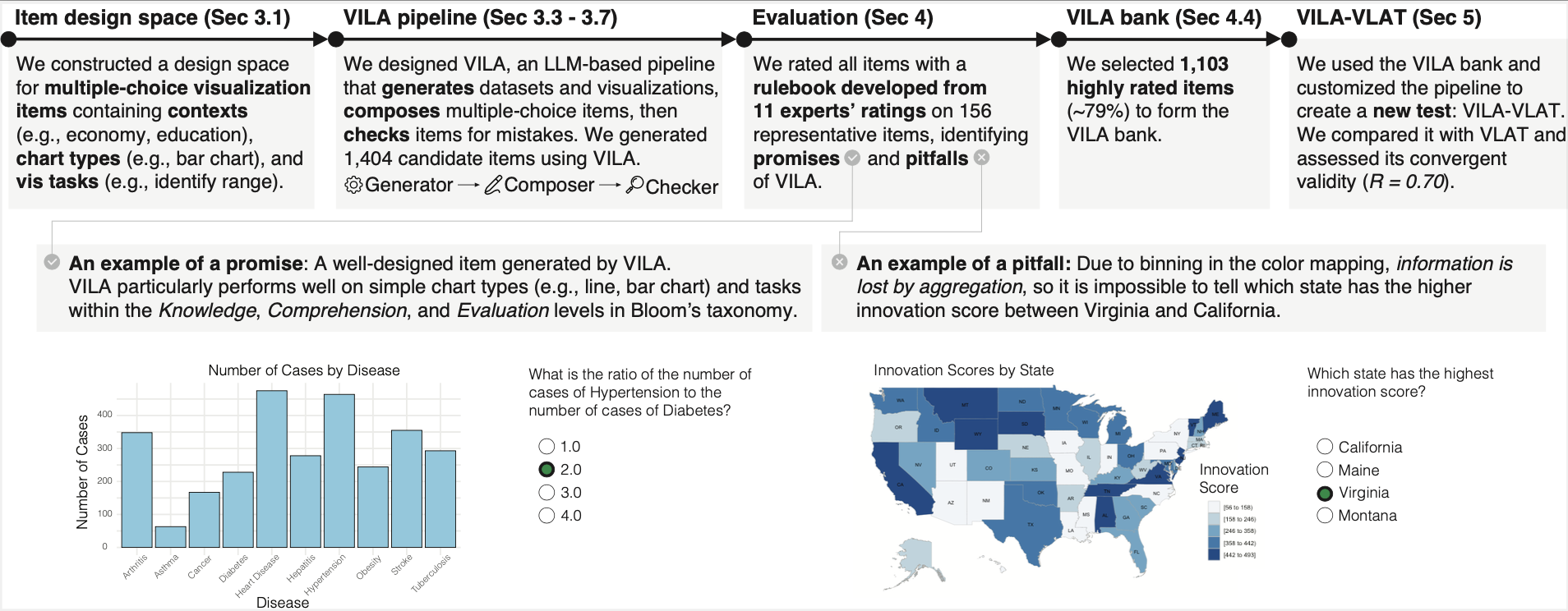
Fast forward
Full Video
Keywords
Visualization Items, Large Language Models, Visualization Literacy Assessment
Abstract
Visualization items—factual questions about visualizations that ask viewers to accomplish visualization tasks—are regularly used in the field of information visualization as educational and evaluative materials. For example, researchers of visualization literacy require large, diverse banks of items to conduct studies where the same skill is measured repeatedly on the same participants. Yet, generating a large number of high-quality, diverse items requires significant time and expertise. To address the critical need for a large number of diverse visualization items in education and research, this paper investigates the potential for large language models (LLMs) to automate the generation of multiple-choice visualization items. Through an iterative design process, we develop the VILA (Visualization Items Generated by Large LAnguage Models) pipeline, for efficiently generating visualization items that measure people’s ability to accomplish visualization tasks. We use the VILA pipeline to generate 1,404 candidate items across 12 chart types and 13 visualization tasks. In collaboration with 11 visualization experts, we develop an evaluation rulebook which we then use to rate the quality of all candidate items. The result is the VILA bank of ∼1,100 items. From this evaluation, we also identify and classify current limitations of the VILA pipeline, and discuss the role of human oversight in ensuring quality. In addition, we demonstrate an application of our work by creating a visualization literacy test, VILA-VLAT, which measures people’s ability to complete a diverse set of tasks on various types of visualizations; comparing it to the existing VLAT, VILA-VLAT shows moderate to high convergent validity (R = 0.70). Lastly, we discuss the application areas of the VILA pipeline and the VILA bank and provide practical recommendations for their use. All supplemental materials are available at https://osf.io/ysrhq/.