Best Paper Award
VisEval: A Benchmark for Data Visualization in the Era of Large Language Models
Nan Chen - Microsoft Research, Shanghai, China
Yuge Zhang - Microsoft Research, Shanghai, China
Jiahang Xu - Microsoft Research, Shanghai, China
Kan Ren - ShanghaiTech University, Shanghai, China
Yuqing Yang - Microsoft Research, Shanghai, China
Download preprint PDF
Download Supplemental Material
Room: Bayshore I + II + III
2024-10-15T16:40:00ZGMT-0600Change your timezone on the schedule page
2024-10-15T16:40:00Z
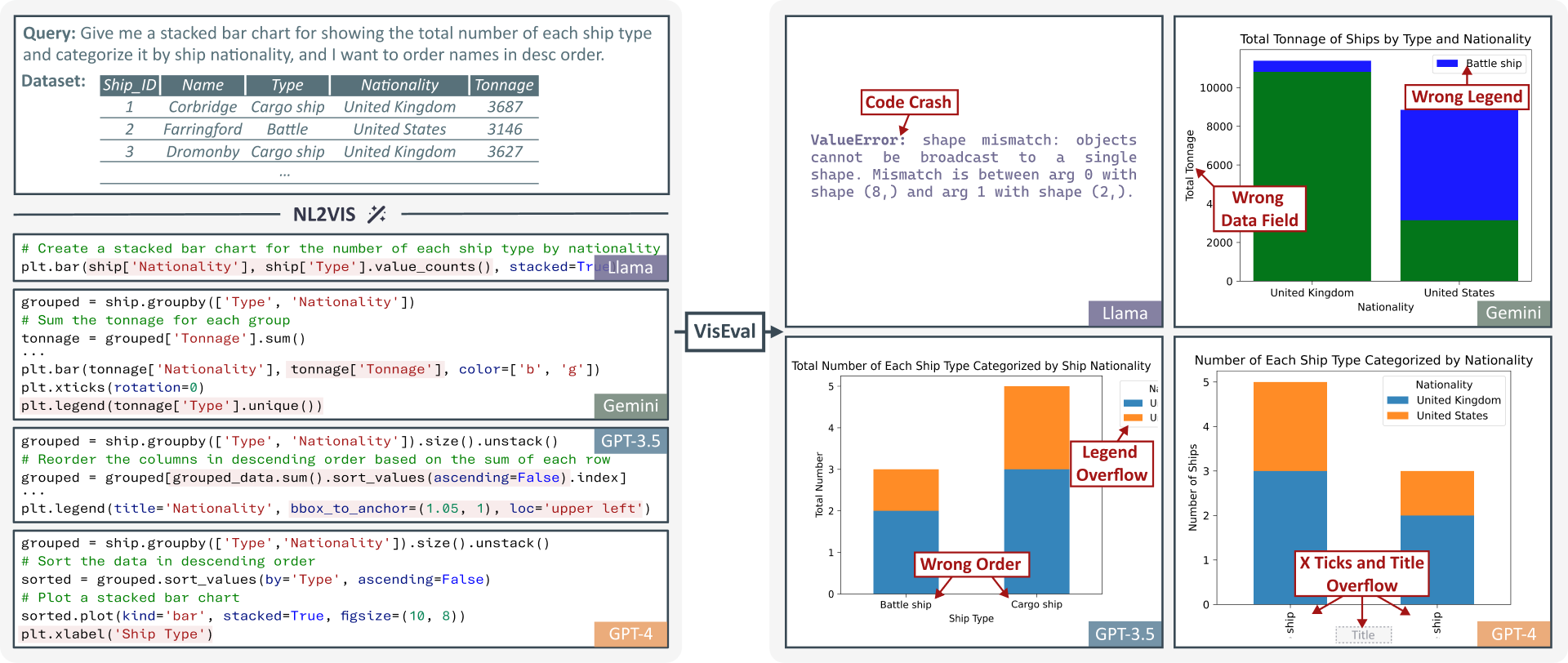
Fast forward
Full Video
Keywords
Visualization evaluation, automatic visualization, large language models, benchmark
Abstract
Translating natural language to visualization (NL2VIS) has shown great promise for visual data analysis, but it remains a challenging task that requires multiple low-level implementations, such as natural language processing and visualization design. Recent advancements in pre-trained large language models (LLMs) are opening new avenues for generating visualizations from natural language. However, the lack of a comprehensive and reliable benchmark hinders our understanding of LLMs’ capabilities in visualization generation. In this paper, we address this gap by proposing a new NL2VIS benchmark called VisEval. Firstly, we introduce a high-quality and large-scale dataset. This dataset includes 2,524 representative queries covering 146 databases, paired with accurately labeled ground truths. Secondly, we advocate for a comprehensive automated evaluation methodology covering multiple dimensions, including validity, legality, and readability. By systematically scanning for potential issues with a number of heterogeneous checkers, VisEval provides reliable and trustworthy evaluation outcomes. We run VisEval on a series of state-of-the-art LLMs. Our evaluation reveals prevalent challenges and delivers essential insights for future advancements.