KMTLabeler: An Interactive Knowledge-Assisted Labeling Tool for Medical Text Classification
He Wang -
Yang Ouyang -
Yuchen Wu -
Chang Jiang -
Lixia Jin -
Yuanwu Cao -
Quan Li -
Screen-reader Accessible PDF
DOI: 10.1109/TVCG.2024.3406387
Room: Bayshore I
2024-10-17T16:00:00ZGMT-0600Change your timezone on the schedule page
2024-10-17T16:00:00Z
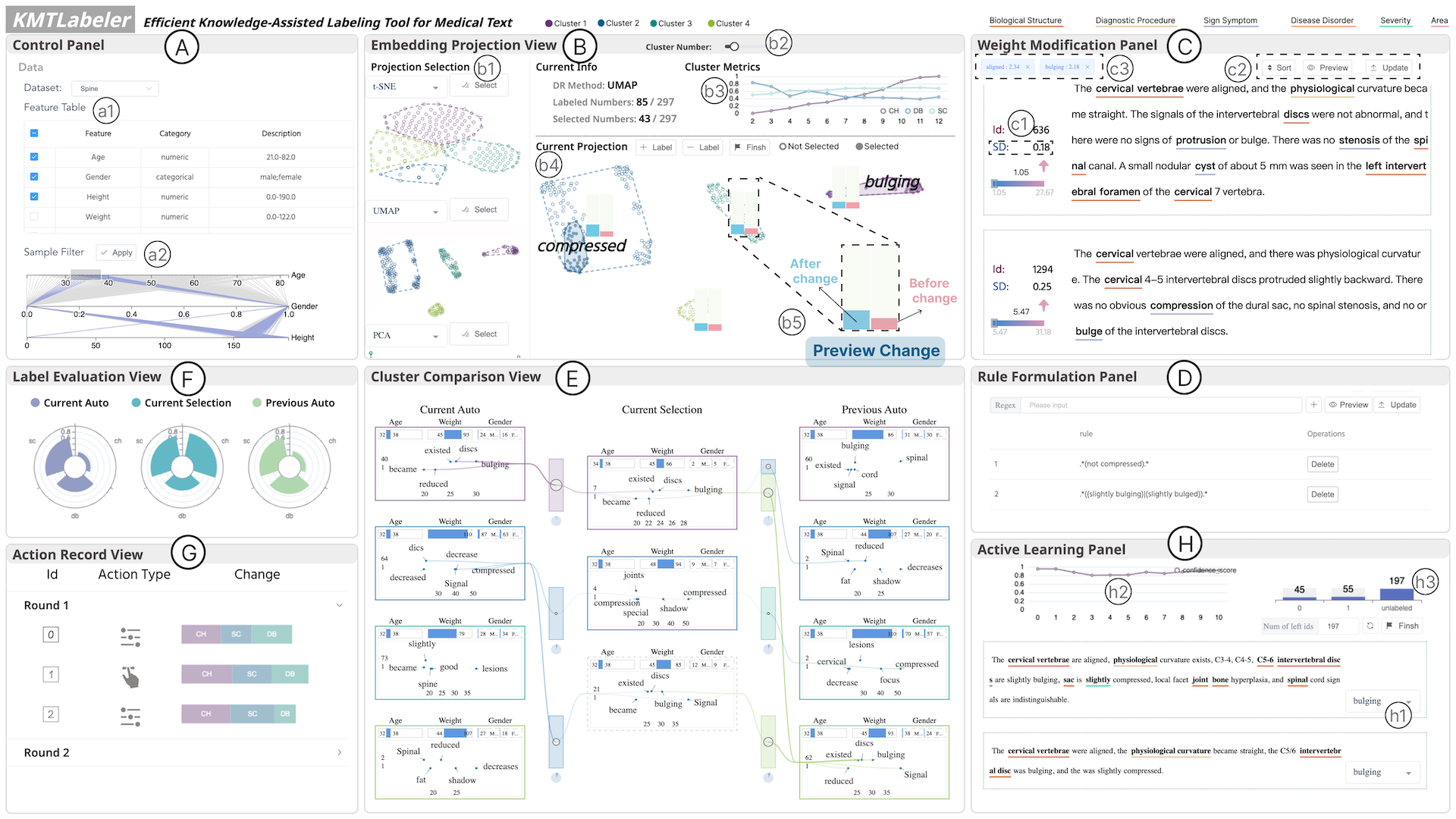
Fast forward
Full Video
Keywords
Medical Text Labeling, Expert Knowledge, Embedding Network, Visual Cluster Analysis, Active Learning
Abstract
The process of labeling medical text plays a crucial role in medical research. Nonetheless, creating accurately labeled medical texts of high quality is often a time-consuming task that requires specialized domain knowledge. Traditional methods for generating labeled data typically rely on rigid rule-based approaches, which may not adapt well to new tasks. While recent machine learning (ML) methodologies have mitigated the manual labeling efforts, configuring models to align with specific research requirements can be challenging for labelers without technical expertise. Moreover, automated labeling techniques, such as transfer learning, face difficulties in in directly incorporating expert input, whereas semi-automated methods, like data programming, allow knowledge integration through rules or knowledge bases but may lack continuous result refinement throughout the entire labeling process. In this study, we present a collaborative human-ML teaming workflow that seamlessly integrates visual cluster analysis and active learning to assist domain experts in labeling medical text with high efficiency. Additionally, we introduce an innovative neural network model called the embedding network, which incorporates expert insights to generate task-specific embeddings for medical texts. We integrate the workflow and embedding network into a visual analytics tool named KMTLabeler, equipped with coordinated multi-level views and interactions. Two illustrative case studies, along with a controlled user study, provide substantial evidence of the effectiveness of KMTLabeler in creating an efficient labeling environment for medical text classification.