Best Paper Award
VADIS: A Visual Analytics Pipeline for Dynamic Document Representation and Information Seeking
Rui Qiu - Ohio State University, Columbus, United States
Yamei Tu - The Ohio State University, Columbus, United States
Po-Yin Yen - Washington University School of Medicine in St. Louis, St. Louis, United States
Han-Wei Shen - The Ohio State University , Columbus , United States
Room: Bayshore I + II + III
Tuesday, October 15th, 2024 @ 16:55GMT+00:00Change your timezone on the schedule page
6 months agoYour current time: Monday, Apr 7th @ 15:14
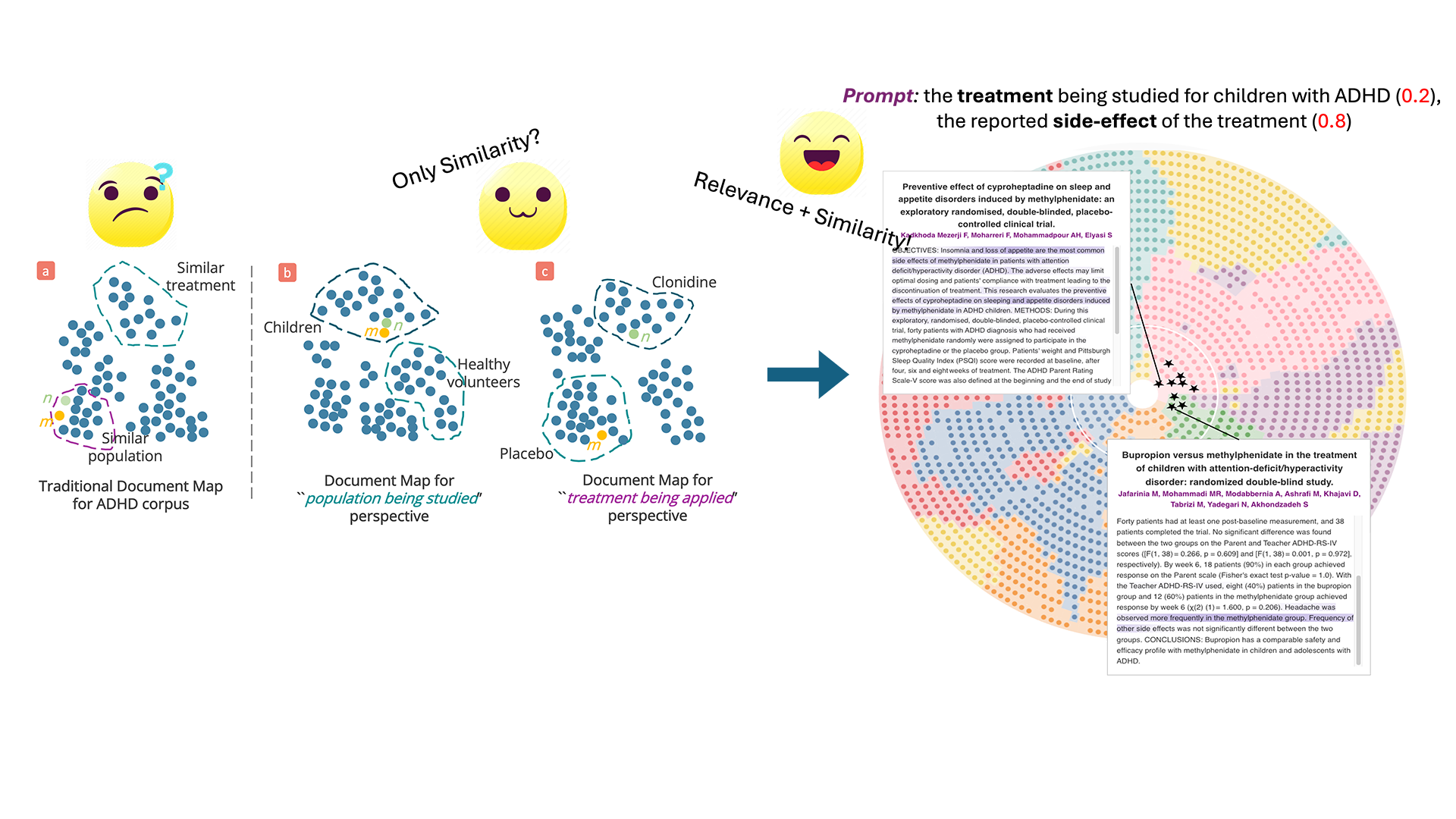
Fast forward
Full Video
Keywords
Attention visualization, dynamic document representation, document visualization, biomedical information seeking
Abstract
In the biomedical domain, visualizing the document embeddings of an extensive corpus has been widely used in information-seeking tasks. However, three key challenges with existing visualizations make it difficult for clinicians to find information efficiently. First, the document embeddings used in these visualizations are generated statically by pretrained language models, which cannot adapt to the user's evolving interest. Second, existing document visualization techniques cannot effectively display how the documents are relevant to users’ interest, making it difficult for users to identify the most pertinent information. Third, existing embedding generation and visualization processes suffer from a lack of interpretability, making it difficult to understand, trust and use the result for decision-making. In this paper, we present a novel visual analytics pipeline for user-driven document representation and iterative information seeking (VADIS). VADIS introduces a prompt-based attention model (PAM) that generates dynamic document embedding and document relevance adjusted to the user's query. To effectively visualize these two pieces of information, we design a new document map that leverages a circular grid layout to display documents based on both their relevance to the query and the semantic similarity. Additionally, to improve the interpretability, we introduce a corpus-level attention visualization method to improve the user's understanding of the model focus and to enable the users to identify potential oversight. This visualization, in turn, empowers users to refine, update and introduce new queries, thereby facilitating a dynamic and iterative information-seeking experience. We evaluated VADIS quantitatively and qualitatively on a real-world dataset of biomedical research papers to demonstrate its effectiveness.