ParamsDrag: Interactive Parameter Space Exploration via Image-Space Dragging
Guan Li - Computer Network Information Center, Chinese Academy of Sciences
Yang Liu - Beijing Forestry University
Guihua Shan - Computer Network Information Center, Chinese Academy of Sciences
Shiyu Cheng - Chinese Academy of Sciences
Weiqun Cao - Beijing Forestry University
Junpeng Wang - Visa Research
Ko-Chih Wang - National Taiwan Normal University
Screen-reader Accessible PDF
Download preprint PDF
Download Supplemental Material
Room: Bayshore I
Wednesday, October 16th, 2024 @ 13:06GMT+00:00Change your timezone on the schedule page
6 months agoYour current time: Monday, Apr 7th @ 15:32
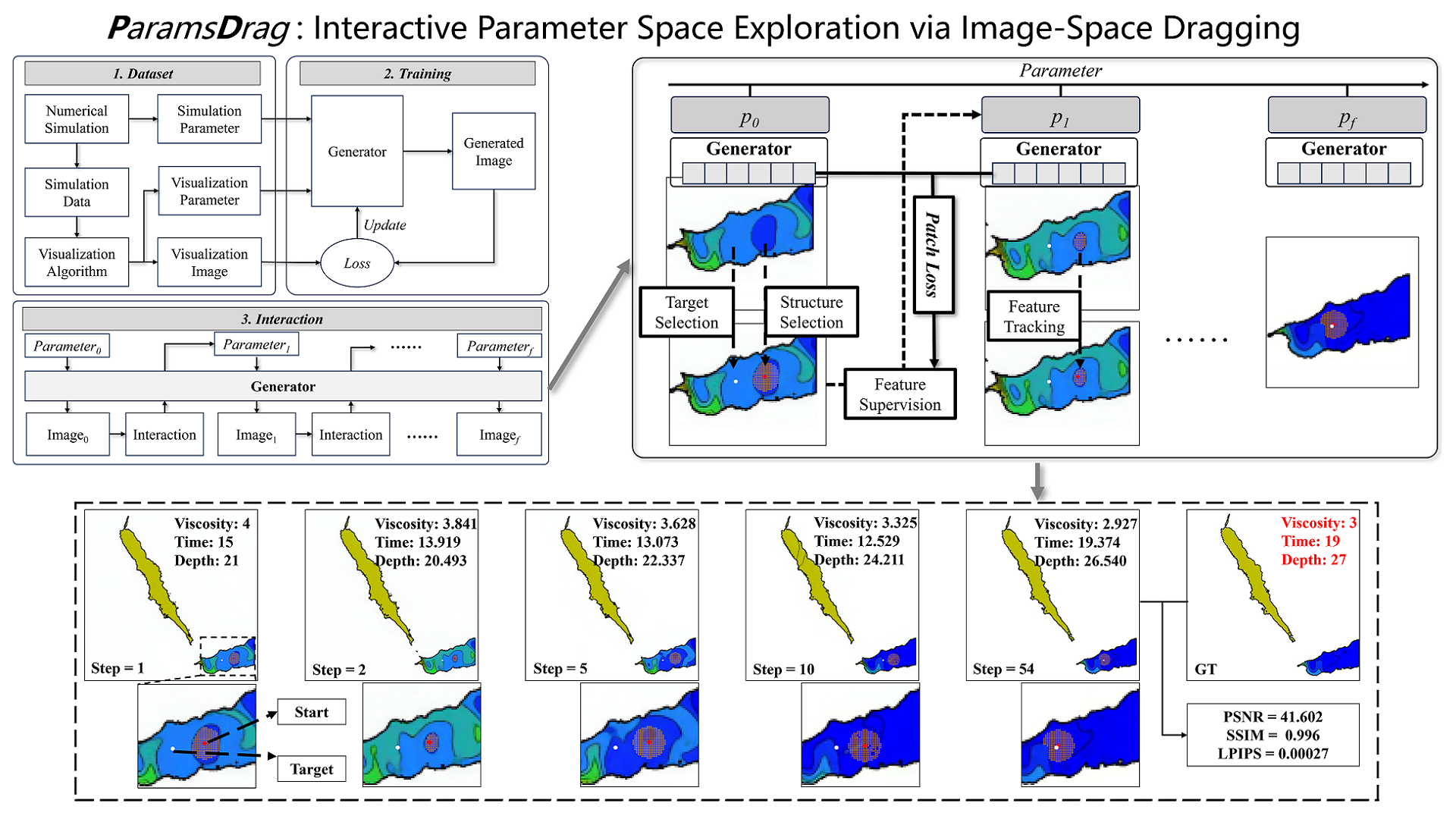
Fast forward
Full Video
Keywords
parameter exploration, feature interaction, parameter inversion
Abstract
Numerical simulation serves as a cornerstone in scientific modeling, yet the process of fine-tuning simulation parameters poses significant challenges. Conventionally, parameter adjustment relies on extensive numerical simulations, data analysis, and expert insights, resulting in substantial computational costs and low efficiency. The emergence of deep learning in recent years has provided promising avenues for more efficient exploration of parameter spaces. However, existing approaches often lack intuitive methods for precise parameter adjustment and optimization. To tackle these challenges, we introduce ParamsDrag, a model that facilitates parameter space exploration through direct interaction with visualizations. Inspired by DragGAN, our ParamsDrag model operates in three steps. First, the generative component of ParamsDrag generates visualizations based on the input simulation parameters. Second, by directly dragging structure-related features in the visualizations, users can intuitively understand the controlling effect of different parameters. Third, with the understanding from the earlier step, users can steer ParamsDrag to produce dynamic visual outcomes. Through experiments conducted on real-world simulations and comparisons with state-of-the-art deep learning-based approaches, we demonstrate the efficacy of our solution.